When it comes to GPUs, you might first think of a graphics card in your computer, and then think of NVIDIA, the world's largest manufacturer of GPU chips. Careful users may have noticed that from May to now, NVIDIA's stock has gone from 30. The dollar rose to $95 and tripled in seven months. Undoubtedly, after many years of deep research in the field of graphics computing, NVIDIA and its GPU products ushered in the best development opportunities in history.
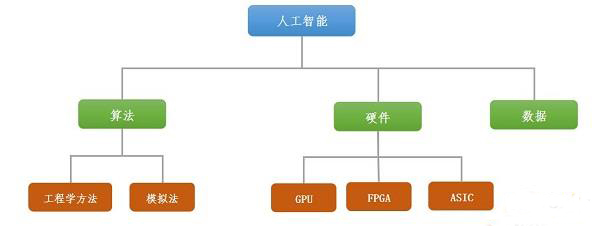
In the past November, IBM and NVIDIA have announced that they will collaborate on the development of a new machine learning tool set, IBM PowerAI, a system that accelerates the training of artificial intelligence and is a software tool that enhances IBM Watson's capabilities. The tool set uses a hybrid hardware architecture of Power processor and NVIDIA GPU. Prior to this, Facebook had already collaborated with NVIDIA on GPUs, and Facebook was the first company to apply NVIDIA's GPUs to the Big Sur artificial intelligence platform, using powerful GPUs and large data sets to build and train artificial intelligence models. In addition, at the 2016 Beijing GTC conference held recently, Baidu artificial intelligence chief scientist Wu Enda also said that Baidu will use GPU for large-scale deployment, consider using GPU to redesign the entire data center, including energy and heat.
On the other hand, Intel, the leader in the CPU field, is also continually adjusting its strategy this year to adapt to the development needs of artificial intelligence. Intel first completed the acquisition of Nervana Systems in August, and Nervana is a supplier of artificial intelligence ASIC chips. The acquisition of Nervana is seen as the first step for Intel against NVIDIA's aggressive attack on artificial intelligence chips. Then, Intel pulled on IBM, Google and Microsoft to plan to form the next generation of artificial intelligence hardware alliance. After Intel acquired Altera and Nervana, it has three major product lines of CPU, FPGA and ASIC to fight against GPU. Intel is blocking the expanding GPU market by integrating these architectures to open up its own artificial intelligence chip strategy. A breakthrough. However, for Intel, in the battle with GPU, the reality is still very cruel.
Since the Internet era, IT has experienced the baptism of the Wintel Alliance for nearly 30 years, making the giants such as Google and IBM recognize the importance of building their own industrial ecosystem. For this reason, no competition for the artificial intelligence chip and hardware industry dominance, any giant can not easily hand over people. Therefore, in addition to planning to form the next generation of artificial intelligence hardware alliance with Intel, Google, Microsoft and IBM are also carrying out their respective industrial layouts.
Microsoft's main approach is to use a specially designed FPGA hardware to support the Azure cloud for cluster computing and learning capabilities. But the problem is that Microsoft, as a software vendor trying to avoid the increasingly mature GPU products, chose to use its own FPGA products to support artificial intelligence applications, regardless of whether the computing power can meet the needs of more and more complex artificial intelligence computing tasks, but only In terms of programming difficulty, the complexity of programming on an FPGA is much higher than that of a GPU platform with a large user base and a mature development environment.
In May of this year, Google announced the development of a dedicated chip TPU for artificial intelligence. This is a special IC designed for deep neural networks. Based on the ASIC architecture, it can be distinguished by neural network through analysis of large amounts of data. Objects, recognition of faces in photos, speech recognition, and text translation. TPU is designed for machine learning, and its calculation accuracy is slightly lower than that of traditional CPU and GPU. Google announced the development of artificial intelligence chip TPU. Its main purpose is to cooperate with the second generation deep learning system TensorFlow launched last year. Build an artificial intelligence ecosystem from hardware to software. TensorFlow is a completely open source free system. Code or programs written in TensorFlow can be used on heterogeneous platforms, from large-scale distributed systems to common mobile phones and tablets. Moreover, when the platform is migrated, the amount of code that needs to be rewritten is very small; from the model test of the laboratory to the product deployment of the product developer, the code seamless connection does not need to be changed.
I believe that Google's launch of TPU in conjunction with TensorFlow may put some pressure on the GPU market, but the overall development of the GPU does not pose a big threat. Of course, if Google's layout is smooth, the future artificial intelligence chip field may form a pattern similar to today's Android and IOS confrontation, but the possibility that TPU completely replaces GPU as the mainstream chip of artificial intelligence is very low.
For IBM, I think IBM will choose to work with NVIDIA on GPUs in the future to enhance the optimization and collaboration between GPU and CPU in the form of a total solution. Since 2004, IBM sold PCs to Lenovo. Over the years, IBM has gradually faded out of the hardware business, and all non-core hardware products have been sold, leaving only core hardware products such as Power products and high-end storage. Re-developing new GPUs with chips, not only the cycle is too long, the input cost is high, and it is not easy to implement. So for now, with Waston as the core, integrating the computing power of GPUs to lay out the artificial intelligence market is IBM's current best choice.
to sum up
I believe that whether it is AISC, FPGA, or CPU, or the architecture integration or integration between these different types of chips, it is not enough to pose a threat to the current development of GPU in the field of artificial intelligence. After so many years of development, GPUs are no longer the traditional graphics processor. In many fields of research and high-performance computing, GPUs have been widely used. The GPU parallel computing architecture is especially suitable for large-scale parallel computing, which can process a large amount of trivial information in parallel. Bai End's chief scientist Wu Enda also said, "In the study of deep learning algorithms, 99% of the computational work can be analogized to multiply different matrices or multiply matrices and vectors, and such calculations are well suited for GPUs. More importantly, after so many years of development, GPUs already have a large user base, as well as relatively mature programming platforms and communities, which provide solid support for the development of GPU in the era of artificial intelligence.
The electric Nursing Bed is used for the treatment and rehabilitation of patients or the elderly. At the beginning, it was mainly used in hospitals. With the development of the economy, electric nursing beds have also entered ordinary households, becoming a new choice for home care, greatly reducing the burden on nursing staff.
Electric nursing bed,in addition to lying flat, the back deck of the bed can be raised and lowered within the range of 0°-80°, and the foot deck can be raised and lowered within the range of 0°-50°. Patients can choose a suitable angle of sitting on the bed to meet the needs of eating, taking medicine, drinking water, washing feet, reading and newspaper, watching TV and moderate physical exercise.
Our selection features - Electric Nursing Bed, Manual Nursing Bed, Nursing Home Bed, Hospital Nursing Bed, etc. We really do have a nursing bed to suit anyone and their requirements.
Electric Adjustable Beds,Hi Lo Homecare Bed,Ultra Low Homecare Bed,Hospital Nursing Care Bed
Jiangmen Jia Mei Medical Products Co.,Ltd. , https://www.jmmedicalsupplier.com